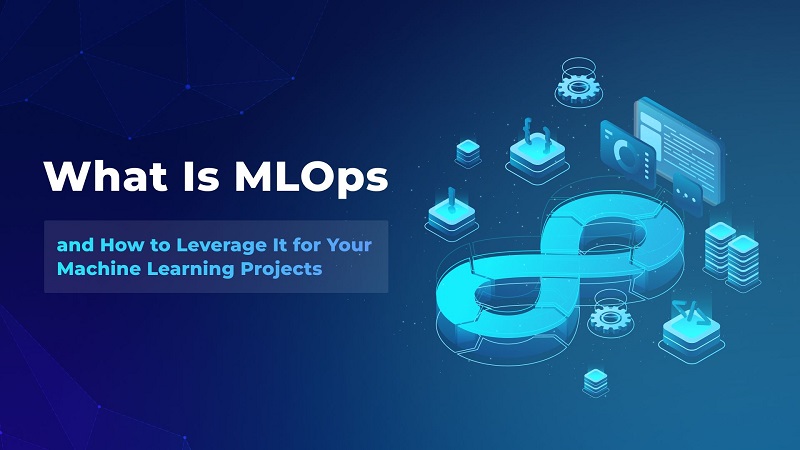
MLOps (Machine Learning Operations) has become a crucial field in the ever-changing field of machine learning, bridging the gap between model creation and practical implementation. The capacity of MLOps to continually monitor model performance, guaranteeing correctness and dependability over time, is one of its most important contributions. This proactive strategy improves business outcomes by assisting businesses in recognizing and resolving problems like model drift and deterioration. Unquestionably, MLOps has an impact on model performance, moving the emphasis from one-time model deployment to continuous optimization.
Monitoring Model Drift: The Secret to Long-Term Accuracy
A significant problem in machine learning is model drift, which happens when the connection between the target variable and the input data varies over time. This may occur as a result of changing market conditions, changing consumer behaviour, or modifications to data collection procedures. To address what is mlops and how does it effect models, MLOps provides the procedures and tools needed to carefully monitor and manage model drift. MLOps solutions are able to identify irregularities and departures from expected behaviour by continuously monitoring key performance indicators (KPIs) such as accuracy, precision, and recall. Data scientists can look into the underlying reasons for drift and take remedial measures before there is a noticeable decline in performance. This proactive strategy guarantees that models continue to produce accurate forecasts and prevents them from becoming outdated.
Retraining Models: Adjusting for Changing Information
MLOps makes it easier to retrain models using new data after model drift has been identified. Retraining entails providing the model with fresh data that represents the environment as it is right now. This effectively corrects the drift by enabling the model to learn the updated relationships between the target variable and the features. When drift is discovered, MLOps workflows may be programmed to start retraining pipelines, guaranteeing that models are updated on a frequent basis.
A Holistic Approach to Optimal Performance
MLOps includes a wider range of procedures that support the best possible model performance, in addition to drift detection and retraining. This covers managing model versions, guaranteeing data integrity, and keeping an eye on data quality. Monitoring data quality makes it easier to spot problems that could impair model accuracy, such as missing values, outliers, and inconsistencies. Version control makes it simpler to revert to earlier iterations when needed by enabling teams to monitor modifications to models and datasets.
Conclusion
MLOps is essential to preserving and enhancing model performance. A successful MLOps plan must include automated retraining, drift detection, continuous monitoring, and comprehensive management of the machine learning lifecycle. Organizations can unlock the full potential of AI by adopting MLOps to guarantee that their machine learning models continue to be accurate, dependable, and in line with business objectives.